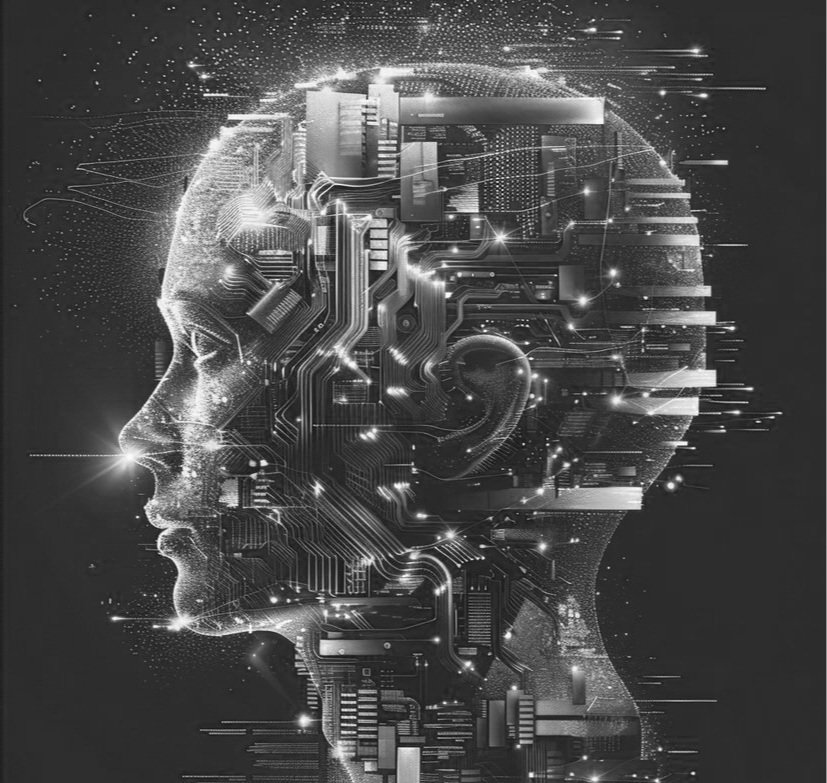
AI Transparency For the Greater Good.
The Transparency Coalition is working to create AI safeguards for the greater good. We believe policies must be created to protect personal privacy, nurture innovation, and foster prosperity.
Fair-minded business and government leaders have created systems to ensure the safety and transparency of the financial industry, the construction industry, the food and drug industries, and the commercial aviation sector. We can and must do the same with artificial intelligence.
Here's where policymakers should start.
-
1. Make it clear when an object is AI-generated
Inform consumers when an image, video, sound, or text has been created or modified by AI. Embed that information in all AI-generated material.
-
2. Publish AI training data ingredients lists
Developers of AI systems should be required to provide documentation for the training data used to develop an AI model. This is as simple as printing an ingredients list on a can of soup.
-
3. Require opt-in consent to use personal data
Flip the paradigm and put people in charge of their personal data. Require consumers to intentionally "opt in" to allow tech companies to collect and use their personal information.
-
4. Minimize the personal data collected and kept
Nobody should have to give up their data privacy just to purchase a pair of socks. Limit data collection to information necessary to perform a transaction or optimize a user's website experience—and nothing more.
1. make it clear when an object is ai-generated
disclose the use of ai.
verify the origin and authenticity of digital content.
The ability to know whether an image, video, sound, or text was created by AI is critical to the healthy functioning of society. Without it we lose two of our most important decision-making tools: The evidence of our eyes and ears. Deepfake videos and other misused AI can cause profound harm to our justice system, medical industry, and electoral process.
A first step is available, and it comes in two parts: embed and disclose.
State lawmakers should require AI developers and deployers to embed provenance data within all digital objects created or modified by a generative AI system. Provenance data is coded within metadata for the purpose of verifying the digital content’s authenticity, origin, or history of modification. Gen AI companies then must offer consumers an easily discovered detection tool on the company’s website. Think of it as a kind of digital decoder ring.
disclosure as a legal baseline
California will require this embed-and-disclose capability beginning on Jan. 1, 2026, thanks to the AI Transparency Act (SB 942) signed recently by Gov. Gavin Newsom. We believe other states should enact similar legislation to give their citizens the ability to sort authentic evidence from machine-made make-believe. State laws requiring AI disclosure tools set a fair and appropriate legal baseline for the tech industry, protecting ethical AI companies from bad actors offering deceptive and malicious products.
the technology is already available
Some leading companies—including Adobe, Nikon, and Microsoft—are already working with a tool known as Content Credentials, which embeds provenance data in a digital object. Clicking a small pin reveals that information, as seen in the example above.
The Content Credentials tool embeds provenance data in a digital object. Clicking the “cr” pin, as in the above image, reveals information about AI use. Disclosure like this should be required of all AI content.
dive deeper
2. Publish AI training data ingredient Lists
this is a data declaration. Post it.
It’s simple and easy. Every AI model should have one.
AI developers should be required to provide documentation for all training data used in the development of an AI model.
This type of auditable information set provides transparency and assurance to deployers, consumers, and regulators. It’s similar to the SOC 2 reports that are standard in the cybersecurity industry. SOC 2 reports, issued by third-party auditors, assess and address the risks associated with software or technology services.
A Data Declaration is not necessarily tied to government oversight. Rather, we believe it should become a standard component of every AI model—expected and demanded by AI system deployers as a transparent mark of quality and legal assurance.
AB 2013: the Emerging standard under state law
In Sept. 2024, Gov. Gavin Newsom signed AB 2013, the California Training Data Transparency Act, into law. This first-in-the-nation state mandate requires AI developers to publish high-level information about the datasets used to train their AI models. The Act, crafted by Assemblymember Jacqui Irwin, marks the first time any legislative body in the United States has adopted a law requiring developers to publicly share the most basic information about an AI model’s training data.
California’s training data disclosures will be required starting on Jan. 1, 2026. Legislators in a number of other states are considering introducing versions of AB 2013 for their jurisdictions in the sessions that open in Jan. 2025. Use the Contact Us form at the bottom of this page to reach a Transparency Coalition expert for more information on activity in your state.
Download our Data Declaration template
The Transparency Coalition has formulated a Data Declaration that would contain basic information about the data used to train the AI model. A number of other data card formulations have been proposed, including Datasets for Datasheets, Data Cards, and Dataset Nutrition Labels. TCAI’s Data Declaration is the only template that is fully compliant with AB 2013 requirements.
FIELD NAME | POSSIBLE VALUES |
---|---|
Data Set Name | Text |
Data Set Owner | Text |
Data Set Description | Text |
Data Set Size | Numerical |
Data Set Category | Web text, images, music, video, books |
Data Set License Type | Commercial License, Proprietary, Public Domain, Fair Use claim |
Data Set License Name | e.g. GPL, Apache, Creative Commons |
Data Set Collection Period | Start date, End date (or Present) |
Data Set Usage Period | Start date, End date (or Present) |
Data Set contains personal or personally identifiable information | Yes or No |
Personal Information Opt-in obtained | Yes or No |
Personal Information License mechanism | EULA, Terms of Service, Privacy Policy, Click Through |
Personal Information anonymized prior to training | Yes or No |
Data Set contains Copyrighted Information | Yes or No |
License governing Copyrighted Information | Fair use, Commercial license |
Synthetic Training Data use | Yes or No |
Dive Deeper
3. Require opt-in consent to use personal data
‘opt-in’ consent is the better way.
want to use my personal data? you’ll need my consent.
Have you noticed all the cookie consent banners popping up nowadays?
Most websites track visitors’ actions using digital markers known as cookies. Cookies are text files stored on a user’s device that track online activity. They’re the little gremlins that allow those annoying ads to follow you around from site to site.
Companies aren’t posting these because they value your privacy. The new consent banners—see example at right—exist only because the European Union and some US states recently enacted laws requiring them.
These laws apply only to cookies, not to the unauthorized collection and use of your personal data to train AI systems. (There is a difference.) They operate on what’s known as an opt-out or opt-in basis.
Opt-out vs. opt-in: a world of difference
Most privacy laws in the United States operate on an opt-out basis, meaning that users are assumed to have consented to the use of cookies unless they actively decline, ie, opt out.
The EU’s General Data Protection Regulation (GDPR), by contrast, is an opt-in mechanism. That means companies may not use personal data unless an individual actively offers consent to do so, ie, opts in.
To comply with laws in both North America and Europe, most companies have created cookie banners like the one pictured because they cover both opt-out (Reject All) and opt-in (Accept All) mechanisms.
using your personal data to train AI systems
With the rise of AI technology, your personal data isn’t just being used to advertise a pair of shoes. Tech companies are scooping up your LinkedIn, Facebook, and YouTube posts and storing them in massive datasets used to train new AI systems. They’re doing it without your consent. They’re making billions of dollars. Your privacy is their product.
Opt-out mechanisms like cookie banners are required for cookies, not personal data. In the U.S. there is no federal or state law requiring companies to offer individuals a way to remove their personal information from the datasets used to train AI systems like ChatGPT.
Cookie consent banners became common only after the EU and some US states passed privacy laws giving consumers the choice to allow or block the tracking devices. Similar laws are needed to give individuals the power to allow or block the collection and use of personal data to train AI models.
it’s simple: Require opt-in consent to use your personal data
Individuals are the rightful owners of their personal data. Companies should assume they can’t collect, store, or use your personal data to train AI models unless you give your affirmative consent. Opt-in should not only be the industry standard; it should be the law of the land.
State legislators can craft those laws right now. And as we see with the cookie banners, it’s easy for companies to post these opt-in banners. But they won’t do it unless we—the people and our elected representatives—require them to.
dive deeper
4. Minimize the personal data collected
gather only necessary data, nothing more.
I just want to buy a shirt.
You don’t need my personal history.
‘Data minimization’ is a privacy principle that limits personal information collected from a consumer to data strictly relevant and necessary to accomplish a specific purpose.
In other words, if you’re ordering a shirt from an online retailer it’s appropriate to collect data about your shirt size, color preference, and mailing address. No shirt seller needs to know your date of birth, gender, racial identity, current geolocation, Social Security number, income level, or employment status.
Data minimization requirements prevent the collection and abuse of personal information before it starts.
key elements of data minimization
Data minimization includes limits on both data collection and data retention:
Collection: Only collect personal information that is directly relevant and necessary to the purpose.
Retention: Only keep the data for as long as it is necessary to fulfill the purpose. No perpetual storage.
examples of data minimization laws
Some US states, as well as the EU, have taken the early lead on setting data minimization requirements.
The California Consumer Privacy Act (CCPA) contains this data minimization clause:
“A business’ collection, use, retention, and sharing of a consumer’s personal information shall be reasonably necessary and proportionate to achieve the purposes for which the personal information was collected or processed, or for another disclosed purpose that is compatible with the context in which the personal information was collected, and not further processed in a manner that is incompatible with those purposes.”
Connecticut, Colorado, Utah, and Virginia have also enacted data minimization requirements as part of their state privacy laws.
data minimization in practice
Data minimization can include policies such as:
Time limitations: Establish retention policies for various data types. For example, retain email data for 90 days, retain employment data every year-end for 5 years.
Establish periodic reviews of retained data: Identify when and how personal information is being deleted or purged.
Establish solutions for deleting personal information upon an individual's request.
If personal information is used as a unique identifier (such as a social security number), consider whether it is possible to use or create an alternate ID.
Dive Deeper
TRANSPARENCY COALITION AI BILL TRACKER
Stay up to date on AI-related bills introduced in the 2024 state legislative session in all 50 states with our interactive map.
Contact Us
If you’re a policymaker or lawmaker and wish to contact us, please fill out this form. We will respond within 24 hours.